RECENT RESEARCH HIGHLIGHTS
Spectral Content of a Single Non-Brownian Trajectory
Time-dependent processes are often analysed using the power spectral density (PSD) calculated by taking an appropriate Fourier transform of individual trajectories and finding the associated ensemble average. Frequently, the available experimental datasets are too small for such ensemble averages, and hence, it is of a great conceptual and practical importance to understand to which extent relevant information can be gained from S(f,T), the PSD of a single trajectory. Here we focus on the behavior of this random, realization-dependent variable parametrized by frequency f and observation time T, for a broad family of anomalous diffusions—fractional Brownian motion with Hurst index H—and derive exactly its probability density function. We show that S(f,T) is proportional—up to a random numerical factor whose universal distribution we determine—to the ensemble-averaged PSD. For subdiffusion (H<1/2), we find that S(f,T)∼ A/f2H+1 with random amplitude A. In sharp contrast, for superdiffusion (H>1/2) S(f,T)∼BT 2H−1/f2 with random amplitude B. Remarkably, for H>1/2 the PSD exhibits the same frequency dependence as Brownian motion, a deceptive property that may lead to false conclusions when interpreting experimental data. Notably, for H>1/2 the PSD is ageing and is dependent on T. Our predictions for both sub- and superdiffusion are confirmed by experiments in live cells and in agarose hydrogels and by extensive simulations.Phys. Rev. X 9, 011019 (2019)
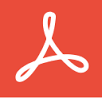
Strong defocusing of molecular reaction times results from an interplay of geometry and reaction control
Textbook concepts of diffusion-versus kinetic-control are well-defined for reaction-kinetics involving macroscopic concentrations of diffusive reactants that are adequately described by rate-constants—the inverse of the mean-first-passage-time to the reaction-event. In contradiction, an open important question is whether the mean-first-passage-time alone is a sufficient measure for biochemical reactions that involve nanomolar reactant concentrations. Here, using a simple yet generic, exactly solvable model we study the effect of diffusion and chemical reaction-limitations on the full reaction-time distribution. We show that it has a complex structure with four distinct regimes delineated by three characteristic time scales spanning a window of several decades. Consequently, the reaction-times are defocused: no unique time-scale characterises the reaction-process, diffusion- and kinetic-control can no longer be disentangled, and it is imperative to know the full reaction-time distribution. We introduce the concepts of geometry- and reaction-control, and also quantify each regime by calculating the corresponding reaction depth.Comm. Chem. 1, 96 (2018)
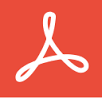
Bayesian nested sampling analysis of single particle tracking data: maximum likelihood model selection applied to stochastic diffusivity data
We employ Bayesian statistics using the nested-sampling algorithm to compare and rank multiple models of ergodic diffusion (including anomalous diffusion) as well as to assess their optimal parameters for in silico-generated and real time-series. We focus on the recently-introduced model of Brownian motion with “diffusing diffusivity”—giving rise to widely-observed non-Gaussian displacement statistics—and its comparison to Brownian and fractional Brownian motion, also for the time-series with some measurement noise. We conduct this model-assessment analysis using Bayesian statistics and the nested-sampling algorithm on the level of individual particle trajectories. We evaluate relative model probabilities and compute best-parameter sets for each diffusion model, comparing the estimated parameters to the true ones. We test the performance of the nested-sampling algorithm and its predictive power both for computer-generated (idealised) trajectories as well as for real single-particle-tracking trajectories. Our approach delivers new important insight into the objective selection of the most suitable stochastic model for a given time-series. We also present first model-ranking results in application to experimental data of tracer diffusion in polymer-based hydrogels.Phys. Chem. Chem. Phys. 20, 29018 (2018)
Brownian yet non-Gaussian diffusion: from superstatistics to subordination of diffusing diffusivities
A growing number of biological, soft, and active matter systems are observed to exhibit normal diffusive dynamics with a linear growth of the mean-squared displacement, yet with a non-Gaussian distribution of increments. Based on the Chubinsky-Slater idea of a diffusing diffusivity, we here establish and analyze a minimal model framework of diffusion processes with fluctuating diffusivity. In particular, we demonstrate the equivalence of the diffusing diffusivity process with a superstatistical approach with a distribution of diffusivities, at times shorter than the diffusivity correlation time. At longer times, a crossover to a Gaussian distribution with an effective diffusivity emerges. Specifically, we establish a subordination picture of Brownian but non-Gaussian diffusion processes, which can be used for a wide class of diffusivity fluctuation statistics. Our results are shown to be in excellent agreement with simulations and numerical evaluations.Phys. Rev. X 7, 021002 (2017)
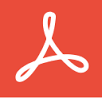