RECENT RESEARCH HIGHLIGHTS
Machine-learning solutions for the analysis of single-particle diffusion trajectories
Modern single-particle-tracking techniques produce extensive time-series of diffusive motion in a wide variety of systems, from single-molecule motion in living-cells to movement ecology. The quest is to decipher the physical mechanisms encoded in the data and thus to better understand the probed systems. We recently proposed machine-learning techniques for decoding anomalous-diffusion data to include an uncertainty estimate in addition to the predicted output. To avoid the Black-Box-Problem a Bayesian-Deep-Learning technique named Stochastic-Weight-Averaging-Gaussian is used to train models for both the classification of the diffusion model and the regression of the anomalous diffusion exponent of single-particle-trajectories. Evaluating their performance, we find that these models can achieve a well-calibrated error estimate while maintaining high prediction accuracies. In the analysis of the output uncertainty predictions we relate these to properties of the underlying diffusion models, thus providing insights into the learning process of the machine and the relevance of the output.Nature Comm. 13, 6717 (2022)
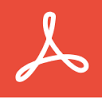
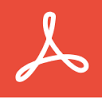
Objective comparison of methods to decode anomalous diffusion
Deviations from Brownian motion leading to anomalous diffusion are found in transport dynamics from quantum physics to life sciences. The characterization of anomalous diffusion from the measurement of an individual trajectory is a challenging task, which traditionally relies on calculating the trajectory mean squared displacement. However, this approach breaks down for cases of practical interest, e.g., short or noisy trajectories, heterogeneous behaviour, or non-ergodic processes. Recently, several new approaches have been proposed, mostly building on the ongoing machine-learning revolution. To perform an objective comparison of methods, we gathered the community and organized an open competition, the Anomalous Diffusion challenge (AnDi). Participating teams applied their algorithms to a commonly-defined dataset including diverse conditions. Although no single method performed best across all scenarios, machine-learning-based approaches achieved superior performance for all tasks. The discussion of the challenge results provides practical advice for users and a benchmark for developers.Nature Comm. 12, 6253 (2021)
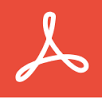
See also Phys. Rev. Res. 4, 033055 (2022)
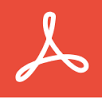
Universal relation between instantaneous diffusivity and radius of gyration of proteins in aqueous solution
Protein conformational fluctuations are highly complex and exhibit long-term correlations. Here, molecular dynamics simulations of small proteins demonstrate that these conformational fluctuations directly affect the protein's instantaneous diffusivity DI. We find that the radius of gyration Rg of the proteins exhibits 1/f fluctuations that are synchronous with the fluctuations of DI. Our analysis demonstrates the validity of the local Stokes-Einstein-type relation DI ∝1/(Rg+R0), where R0∼0.3 nm is assumed to be a hydration layer around the protein. From the analysis of different protein types with both strong and weak conformational fluctuations, the validity of the Stokes-Einstein-type relation appears to be a general property.Phys. Rev. Lett. 126, 128101 (2021)
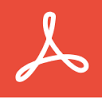
Spectral Content of a Single Non-Brownian Trajectory
Time-dependent processes are often analysed using the power spectral density (PSD) calculated by taking an appropriate Fourier transform of individual trajectories and finding the associated ensemble average. Frequently, the available experimental datasets are too small for such ensemble averages, and hence, it is of a great conceptual and practical importance to understand to which extent relevant information can be gained from S(f,T), the PSD of a single trajectory. Here we focus on the behavior of this random, realization-dependent variable parametrized by frequency f and observation time T, for a broad family of anomalous diffusions- fractional Brownian motion with Hurst index H - and derive exactly its probability density function. We show that S(f,T) is proportional - up to a random numerical factor whose universal distribution we determine - to the ensemble-averaged PSD. For subdiffusion (H<1/2), we find that S(f,T)∼ A/f2H+1 with random amplitude A. In sharp contrast, for superdiffusion (H>1/2) S(f,T)∼BT 2H-1/f2 with random amplitude B. Remarkably, for H>1/2 the PSD exhibits the same frequency dependence as Brownian motion, a deceptive property that may lead to false conclusions when interpreting experimental data. Notably, for H>1/2 the PSD is ageing and is dependent on T. Our predictions for both sub- and superdiffusion are confirmed by experiments in live cells and in agarose hydrogels and by extensive simulations.Phys. Rev. X 9, 011019 (2019)
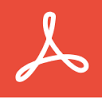
Enhancer-insulator pairing reveals heterogeneous dynamics in long-distance 3D gene regulation
Cells regulate fates and complex body plans using spatiotemporal signaling cascades that alter gene expression. Short DNA sequences, known as enhancers (50-1500 base pairs), help coordinate these cascades by attracting regulatory proteins that enhance the transcription by binding to distal gene promoters. In humans, there are hundreds of thousands of enhancers dispersed across the genome, which poses a challenging coordination task to prevent unintended gene activation. To mitigate this problem, the genome contains insulator elements that block enhancer-promoter interactions. However, there is an open problem with how the insulation works, especially as enhancer-insulator pairs may be separated by millions of base pairs. Based on recent empirical data from Hi-C experiments, this paper proposes a new mechanism that challenges the common paradigm that rests on specific insulator-insulator interactions. Instead, this paper introduces a stochastic looping model where insulators bind weakly to chromatin rather than other insulators. After calibrating the model to experimental data, we use simulations to study the broad distribution of hitting times between an enhancer and a promoter when insulators are present. We find parameter regimes with large differences between average and most probable hitting times. This makes it difficult to assign a typical timescale and hints at highly defocused regulation times. We also map our computational model onto a resetting problem that allows us to derive several analytical results. Besides offering new insights into enhancer-insulator interactions, our paper advances the understanding of gene regulatory networks and causal connections between genome folding and gene activation.Phys. Rev. X Life 2, 033008 (2024)
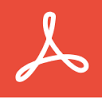